How to compare 2 data sets?
Added on 19/08/2022
Do you understand the potential impact of using 2 independent datasets? In this blog, we provide you a taste of some basic insights.
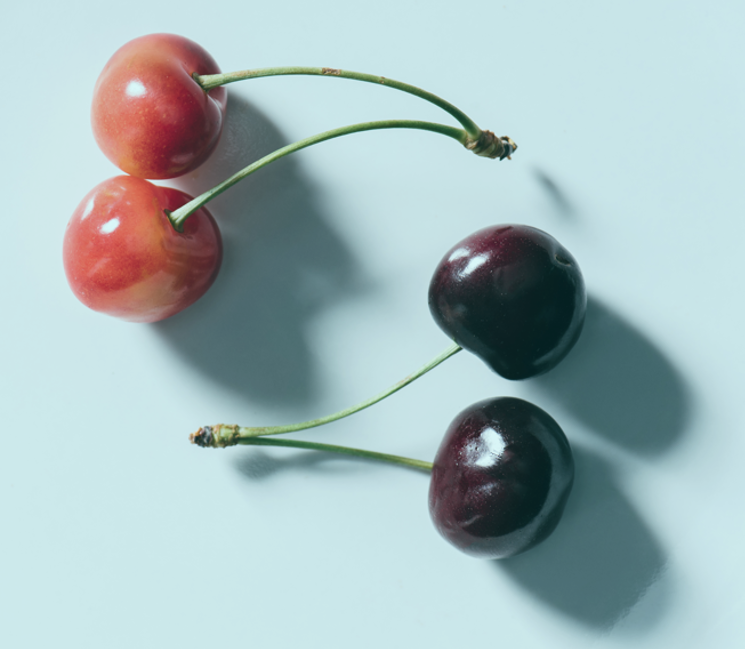
In our former blog, we stipulated the need for reliable clinical data when comparing 2 interventions. But, how to define ‘reliable clinical data’? Even when data are scientifically correct the way they are used in comparisons can reduce the reliability of the results. No need to say that decision makers prefer to see an analysis made based on comparable data sets before (conditional) market access will be granted (or not).
A good example is the variability of treatment duration. When you search for data (treatment duration) a first wave of happiness will appear when data are traced you are looking for. But when examining these data a bit more in detail some elements can cause some frustration. Our recommendation is to look twice (or more) to the published data before using them in the models and reports.
Are the data expressed as mean or as median values? Our recommendation is to strive for mean data. Unfortunately, most publications only present median data. Median data can be used provided that a sufficient number of patients is included in the study and that the study is mature enough. Median values for treatment duration are usually available as from the first analysis on. However, the median value only considers a subset of values; it discards all data except for the most central value(s). The values of the data not taken into account for the determination of the median can strongly influence the mean and can steer the results in a completely different way.
A second point of attention is the scaling. Is the treatment duration expressed in time (weeks, months or years) or in cycles? Over the past years comparisons have been made based on non-matched scaling (e.g. months were used for the innovator versus cycles of 28 days for the comparator). In such case, one needs to ‘translate’ at least one of the datasets to proceed in a uniform way.
The definition of ‘time on treatment’ should also be carefully considered. Some treatment options allow to treat the patients over a number of cycles followed by a treatment interruption (treatment holiday) after which the treatment continues (or not). A typical problem for health economists is to make the decision how the drug cost can be captured most correctly over time in the model. One can calculate the cost per treatment cycle after which the average cost per week (or day) is calculated or the model can be programmed so that the model perfectly mimics the ‘real life situation’ (example: 4 weeks ‘on’ followed by 2 weeks ‘off’). To reduce complexity, we recommend to proceed with a weighted cost per model cycle. This should include the effect of dose reduction(s) or up-titration(s) as well the effect of drug adherence (patients compliance).s
Bear in mind that ‘time on treatment’ (ToT) is different versus ‘time to progression’ (TTP). A treatment can be given in line with the label (and/or reimbursement criteria) which restricts the number of cycles allowed (e.g. 6 cycles typically seen for chemotherapeutics or 24 months for some immunotherapies). The analysis should include the allowed posology as reliable as possible (in line with the study data) while avoiding model complexity.
When head to head studies are lacking, an indirect comparison method will be used, comparing two independent datasets . Always think about the similarity of the data; it doesn’t make sense to compare a dataset from a population which includes paediatrics versus a dataset from an adult population. You will have to select out the paediatrics before you continue the comparison. Of course, reducing the patient numbers will impact the robustness of the data from the patients left in the dataset (a larger confidence interval can be expected). And… do you have access to the data you need from the selected cohort? Also in this case, always interact with the clinical experts and involve an expert in biostatistics before you claim the ‘eureka’ moment.
Even when the treatment duration/number of treatment cycles is limited, the treatment effect can persist for some time after the treatment is stopped or interrupted, impacting the efficacy results over time. Be sure that the extrapolations made are clinically relevant and reliable and avoid cherry picking or extrapolations based on gut feeling. The potential sustained effect after treatment discontinuation should always be validated with clinical experts. When a study does not include an adequate follow-up period to assess this effect, we recommend to avoid integrating it in the base case.
How to define progression free survival? Is some dossiers, ‘progression’ is not clearly defined. In general, progression is measured via diagnostic imaging (tumour size) and/or defined based on biomarkers. Both the innovation and the selected comparator should of course use the same methodology. If the measure of progression free survival of your innovation is based on the value of a tumour marker and the competitor is using radiographic progression, this can not be compared without clinical validation.
A tricky variable is related to combination treatments. The treatment duration as well as the cycle length of the molecules given can differ. This should be projected as correctly as possible in the model. In parallel, a modeller should think about the available options to include the costs of drug administration (intravenous injections) and the costs due to drug toxicity and adverse events. It is almost impossible to identify which molecule has caused the safety event. Always interact with clinical experts and programme the model as such that the user can trace the impact of different model settings.
All of the examples above are related to treatment duration but, no need to say that ‘data collection’ is also related to all the variables as used in the simulation models. Always align the selected data as much as possible and validate the final dataset with clinical experts. Of course, in some cases, it is not possible to align the data from your innovator with the data from the comparator. Also in this case, be open and transparent about the data used and inform decision makers proactively. This way, we believe that you can optimise the value of innovation.