Quality of Life – be vigilant!
Added on 16/09/2021
Quality of life (QoL) in our field of expertise is expressed in ‘utilities’. When performing health economic assessments, the delta utility between 2 clinical settings is measured. Be vigilant, though: the way the QoL is assessed in your study needs to be analysed carefully. In our blog we address some potential difficulties you may encounter.
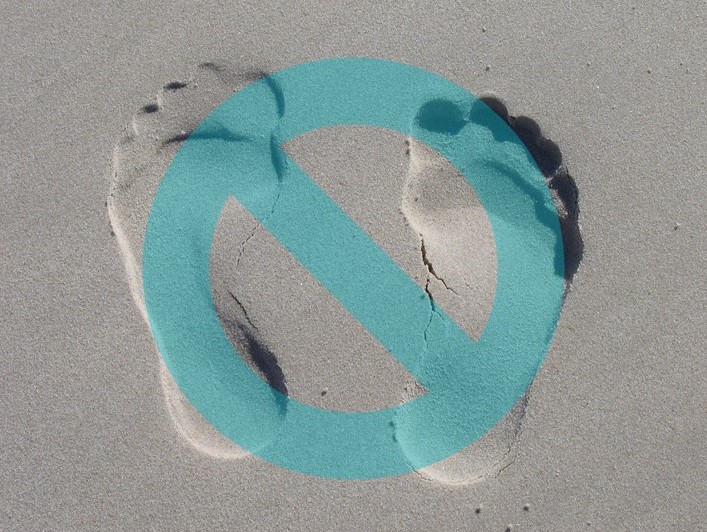
Δ = innovation – SoC
In general, in the world of market access of health care products, quality of life (QoL) is expressed in ‘utilities’. The healthier you are, the higher your utility score is. When performing health economic assessments, the difference in utilities for patients (delta utility) between the situation without and the situation with the innovation. The starting point is the QoL of the patient diagnosed and treated with ‘standard of care’, which is compared with the QoL dataset of patients being treated (or tested) by means of an innovation.
Many better than 1
Although in theory this is a very nice instrument, the actual utility-value of an individual patient can be very volatile over time (due to patient characteristics changing, treatment effects, external factors, etc.). In order to make utilities a more robust and thereby robust tool, one needs to estimate the utility not based on just 1 patient but on a population sample that is big enough to be representative for the ‘average patient’. In general, the study population of the clinical trial is used as sample population. By measuring the utilities over time (preferably at predefined timepoints) in both subgroups (of which 1 subgroup represents the ‘standard of care’ and the other the population subjected to your innovation) one can estimate the ‘delta’ between both.
Uncertainty
Some studies are designed to measure utilities in both subgroups of the study (example: from patients with more severe disease ). But when these (subgroup-)data are not available, another solution is needed. Of course, this will cause uncertainty. Optionally, you can rely on data from literature, but this will generate additional uncertainty as the population of interest very likely does not match completely with your study population. To make matters worse, utilities will most likely not have been measured the same way.
Time horizon
Let’s assume you have 2 data sets with all the utility information you want. A new hurdle may appear: for how long will the difference in QoL be maintained?
An example. When you feel acute pain, you have several options: you can accept the situation, take no medication and the rest of your day will be a bit of an off-day. Or you swallow a tablet that reduces the pain over time. Having taken your painkiller will have improved your quality of life for a few hours. Acute pain in general, however, is of a temporary nature and the medication was also meant for a short-term effect. In this particular case, your health state will return to ‘baseline’ and the resulting QoL will be comparable with the QoL you had before experiencing pain. If we now calculate the difference in QoL over a life-time horizon (which hopefully is very long and without pain), the delta effect caused by taking pain medication once will be negligible. But when the health economic study would be done over a very short time horizon, the effect would be much more impressive and the investment done (the cost of the pain medication) would be well balanced with the benefits (a day with less or no pain).
Pain can be chronic as well, and some patients may need long-term treatment. In that case, it makes more sense to apply a long-term horizon in the health economic study. Our recommendation is to apply a time horizon that at least includes the duration of the treatment effect completely.
Liberate true QoL gains from your data
In health economic models, the time horizon may be set to ‘end of life’ almost by default. Occasionally, this causes the delta in the effect to be extrapolated over time where only short-term data were available. And sometimes the delta effect is expressed as an absolute delta which is kept unchanged over time. In almost all health economic studies, the simulation is not completely in line with ‘real life’. When a life-time horizon is selected, one can assume that elderly people will consume more healthcare support than younger people. And that people’s quality of life will decrease over the years, separate from any loss of QoL caused by their disease. The improvement in their QoL during their treatment may get diluted over time and sometimes gets overruled by new diseases and lifetime events. This aspect is often poorly captured or even completely absent in some health economic studies, thereby obscuring the true gains in QoL.
Appropriate background comparison
We agree, most of the models correct for disutilities over a lifetime by taking the ‘average population’ in the country of interest (background utilities). But, does this make sense for all diseases? Patients with (long term) effects due to e.g. stroke, amputation, chronic migraine or diabetes cannot be set equal to the ‘average population’. And adding an absolute delta utility due to the ‘treatment’ (even when treated long time ago) should be analysed with sufficient gut .
Therefore, the impact on the QoL as included in health economic studies needs to be analysed carefully and validation is recommended.
Do you have questions or do you want to get in touch with us:
Your Hebias team.