Clinical data, the key to a good market access dossier
Added on 07/07/2021
Before you introduce an innovation, you will collect data on a relatively limited scale, after which you will compare this to a similar dataset. But how can you make a good comparison and which information can you take into account?
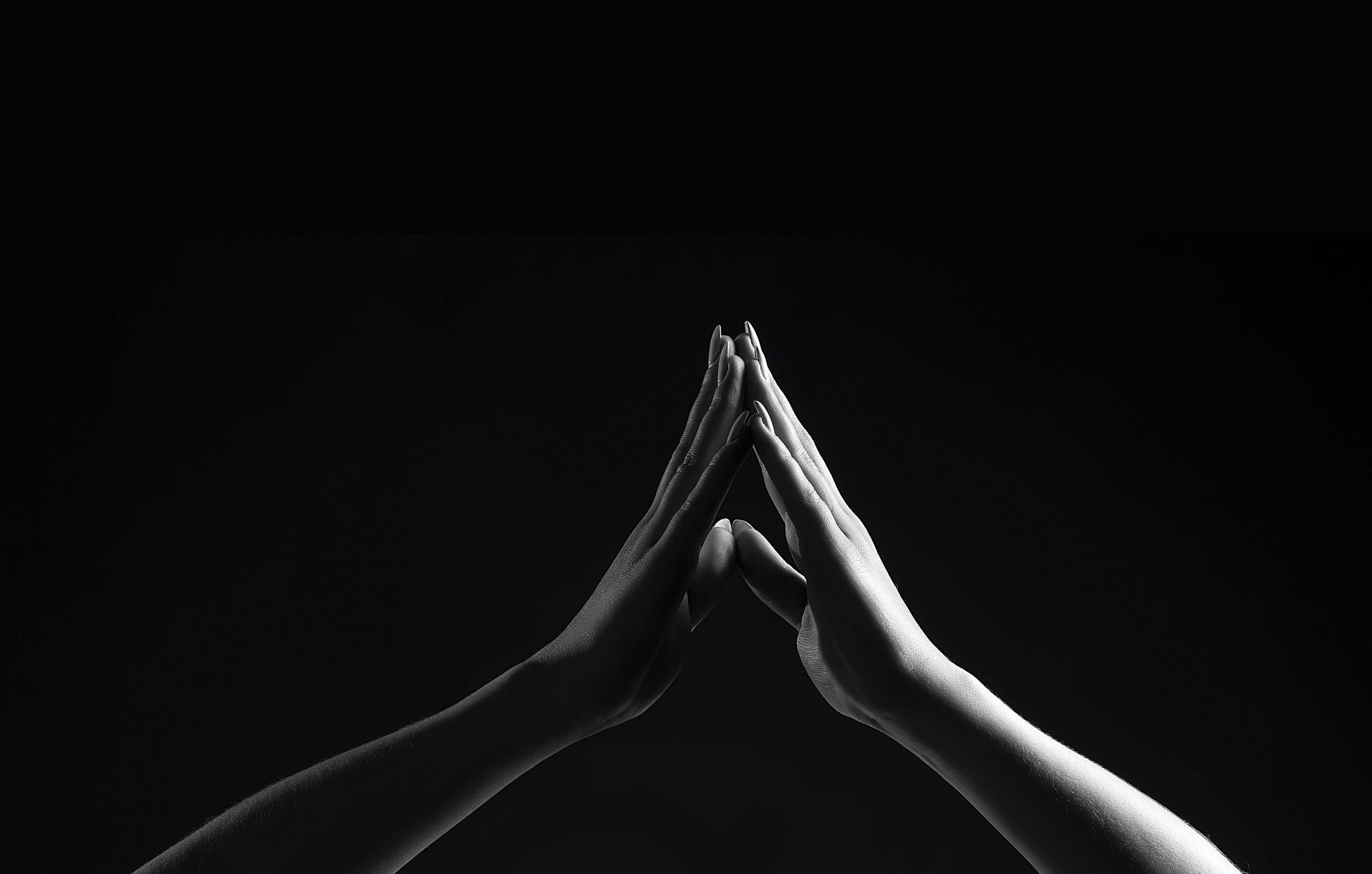
The chicken or the egg. It’s a question that keeps many busy. The budget can be synonymous with the chicken and innovations synonymous with the egg (or the other way around, of course …). Anyway, any kind of innovation results in shifts in resources (lower consumption, higher efficacy, fewer side effects). For pharmaceuticals, it is up to the developers to predict this (expected) shift, after which assessors review this and the payors decide whether the costs/benefits are balanced.
Within healthcare, innovation is usually introduced on the basis of relatively small-scale samples, after which extrapolation(s) estimate what the effect can be within a population (your target group). Additional data may be collected by following patients into the future (prospectively) or by looking back at already generated data (retroactively).
Either way, before you introduce an innovation, you will collect data on a relatively limited scale, after which you will compare this to a similar dataset. Ideally, a clinical trial has been designed containing at least 2 comparable cohorts. For example, one group can be treated using your innovation and the comparison group with the ‘standard of care’. If the study does not include a comparative group (‘arm’), then an indirect comparison will have to be made.
Direct comparative studies normally result in similar datasets where the delta effect can be determined. However, you need to critically analyse the 2 (or more) datasets before you compare them. For example, the cohorts treated in your study can be relatively comparable (age, gender, race, etc.), yet deviations may occur due to external factors (level of expertise of treating physician, guidelines, financial resources, …). In other words, you should know the dataset from your study and possibly adjust it by excluding certain patients or entire subgroups. Setting up a good study protocol including market access considerations is key and increases the usability of the collected data.
Limited financial resources, few patients, a broad goal (e.g., focused on multiple indications) can lead to the choice to set up the study without a comparative arm. This will result in basically a large dataset from one pool. But how do you compare such data with current clinical practice? In other words, you have to indirectly compare with data extracted from another study or studies. Indirect comparisons, however, are not the most optimal. Multiple parameters in that study population will most likely be different (e.g., different ratio in terms of gender, different average age, environmental factors, different inclusion and exclusion criteria, etc.). In the final step, you will have to ensure that the study data are as similar as possible: you have to ‘match’ them. Within health economics, over the last decade, more and more use has been made of ‘matching-adjusted indirect comparisons’ (MAIC). In order to make the dataset of your study more comparable to the data from the other study, the datasets are reduced. But while the datasets are getting better matched, your dataset is getting smaller (and therefore statistically less reliable).
The challenge is therefore to keep the robustness of your study data as high as possible, but also to get the reliability of the comparable dataset as optimal as possible. Biostatisticians know how to do this, and use scientifically sound statistical techniques to obtain the best possible ‘comparable’ dataset. It is advisable to look at your dataset critically and discuss it in your file in all transparency. This will support the assessor (the payor) in assessing the true added value of your innovation, whilst understanding the uncertainties. In this way, you can jointly define an optimal cost/benefit balance that benefits both and this with your primary goal in mind: to bring innovation to the user (usually the patient) who actually benefits from the innovation in question. In this way, the quality of life and/or life expectancy can increase within the intended target group thanks to the efforts made at the start.
Do you have questions about how to set-up an effective to-the-point study protocol that will address all your future market access needs and/or would you like to know the potential risks that may arise? We are happy to support you in setting up your analysis.
See you soon,
Your HEBIAS team.